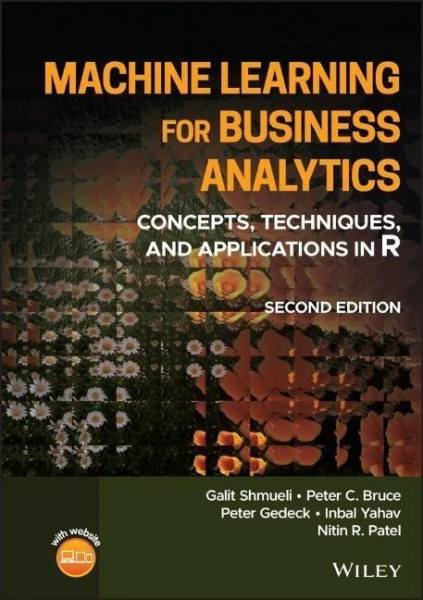
Machine Learning for Business Analytics
Kurzinformation



inkl. MwSt. Versandinformationen
Artikel zZt. nicht lieferbar
Artikel zZt. nicht lieferbar

Beschreibung
MACHINE LEARNING FOR BUSINESS ANALYTICS Machine learning --also known as data mining or data analytics-- is a fundamental part of data science. It is used by organizations in a wide variety of arenas to turn raw data into actionable information. Machine Learning for Business Analytics: Concepts, Techniques, and Applications in R provides a comprehensive introduction and an overview of this methodology. This best-selling textbook covers both statistical and machine learning algorithms for prediction, classification, visualization, dimension reduction, rule mining, recommendations, clustering, text mining, experimentation, and network analytics. Along with hands-on exercises and real-life case studies, it also discusses managerial and ethical issues for responsible use of machine learning techniques. This is the second R edition of Machine Learning for Business Analytics. This edition also includes: * A new co-author, Peter Gedeck, who brings over 20 years of experience in machine learning using R * An expanded chapter focused on discussion of deep learning techniques * A new chapter on experimental feedback techniques including A/B testing, uplift modeling, and reinforcement learning * A new chapter on responsible data science * Updates and new material based on feedback from instructors teaching MBA, Masters in Business Analytics and related programs, undergraduate, diploma and executive courses, and from their students * A full chapter devoted to relevant case studies with more than a dozen cases demonstrating applications for the machine learning techniques * End-of-chapter exercises that help readers gauge and expand their comprehension and competency of the material presented * A companion website with more than two dozen data sets, and instructor materials including exercise solutions, slides, and case solutions This textbook is an ideal resource for upper-level undergraduate and graduate level courses in data science, predictive analytics, and business analytics. It is also an excellent reference for analysts, researchers, and data science practitioners working with quantitative data in management, finance, marketing, operations management, information systems, computer science, and information technology. von Shmueli, Galit (National Tsing Hua University, Taiwan);Bruce, Peter C. (Massachusetts Institute of Technology, USA);Gedeck, Peter (UVA School of Data Science, USA);Yahav, Inbal (The Coller School of Management at Tel Aviv University, Israel);Patel, Nitin R. (Cytel Inc);
Produktdetails
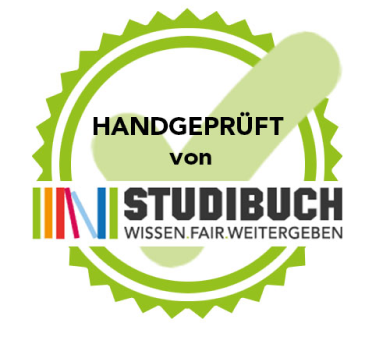
So garantieren wir Dir zu jeder Zeit Premiumqualität.
- hardcover
- 240 Seiten
- Erschienen 2022
- Wiley
- Kartoniert
- 768 Seiten
- Erschienen 2021
- mitp
- Hardcover
- 256 Seiten
- Erschienen 2022
- Wiley & Sons
- Kartoniert
- 180 Seiten
- Erschienen 2020
- UTB GmbH
- Hardcover
- 288 Seiten
- Erschienen 2015
- Wiley
- Gebunden
- 611 Seiten
- Erschienen 2021
- SAP PRESS
- Hardcover -
- Erschienen 2023
- Wiley John + Sons
- Kartoniert
- 68 Seiten
- Erschienen 2022
- Diplomica Verlag
- Hardcover
- 256 Seiten
- Erschienen 2023
- Wiley & Sons