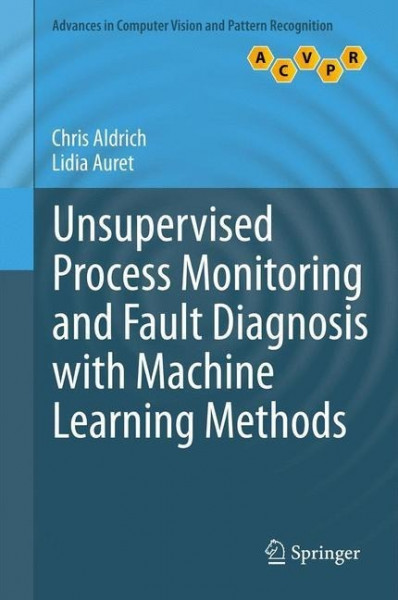
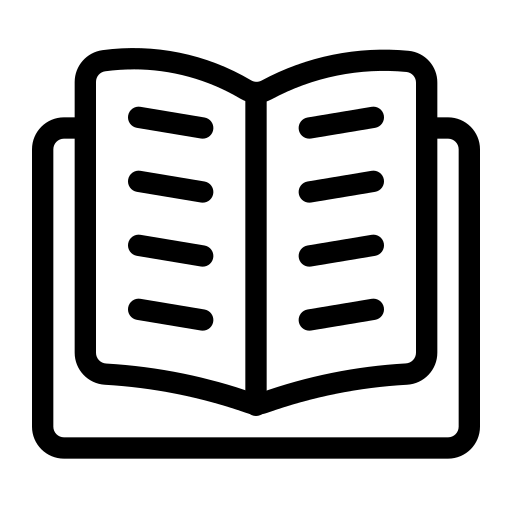
Unsupervised Process Monitoring and Fault Diagnosis with Machine Learning Methods
Kurzinformation



inkl. MwSt. Versandinformationen
Artikel zZt. nicht lieferbar
Artikel zZt. nicht lieferbar

Beschreibung
This unique text/reference describes in detail the latest advances in unsupervised process monitoring and fault diagnosis with machine learning methods. Abundant case studies throughout the text demonstrate the efficacy of each method in real-world settings. The broad coverage examines such cutting-edge topics as the use of information theory to enhance unsupervised learning in tree-based methods, the extension of kernel methods to multiple kernel learning for feature extraction from data, and the incremental training of multilayer perceptrons to construct deep architectures for enhanced data projections. Topics and features: discusses machine learning frameworks based on artificial neural networks, statistical learning theory and kernel-based methods, and tree-based methods; examines the application of machine learning to steady state and dynamic operations, with a focus on unsupervised learning; describes the use of spectral methods in process fault diagnosis. von Aldrich, Chris und Auret, Lidia
Produktdetails
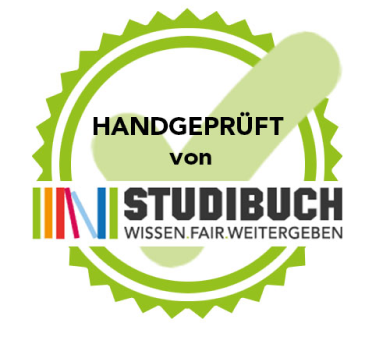
So garantieren wir Dir zu jeder Zeit Premiumqualität.
Über den Autor
- Hardcover
- 256 Seiten
- Erschienen 2022
- Wiley & Sons
- Hardcover
- 388 Seiten
- Erschienen 1993
- Springer
- Gebunden
- 738 Seiten
- Erschienen 2011
- Springer
- Hardcover -
- Erschienen 2017
- Springer
- Kartoniert
- 768 Seiten
- Erschienen 2021
- mitp
- Hardcover
- 256 Seiten
- Erschienen 2023
- Wiley & Sons
- Kartoniert
- 180 Seiten
- Erschienen 2020
- UTB GmbH
- Hardcover
- 624 Seiten
- Erschienen 2007
- Springer
- Taschenbuch
- 218 Seiten
- Erschienen 2012
- Morgan & Claypool Publishers
- Hardcover
- 432 Seiten
- Erschienen 2023
- Wiley & Sons